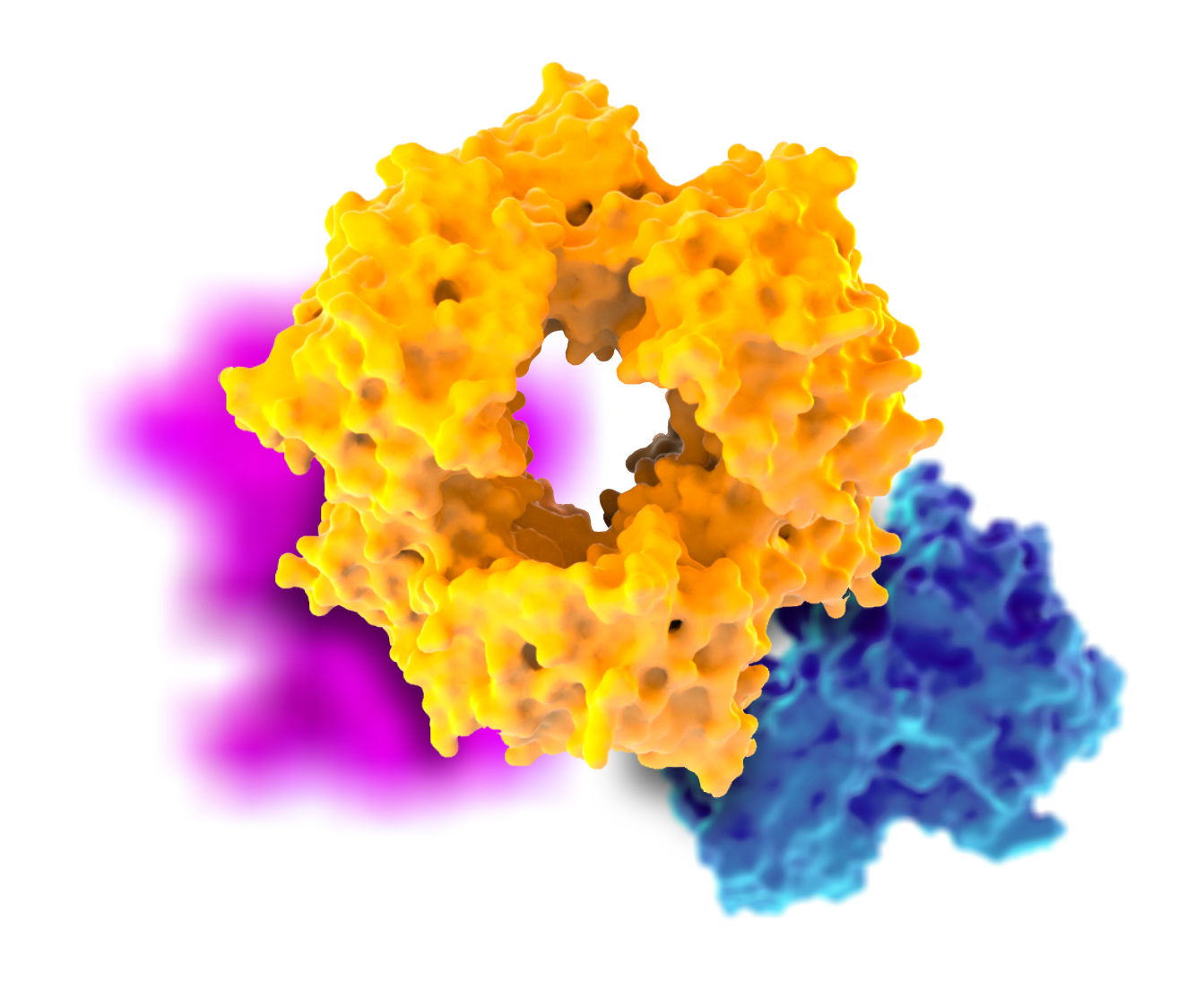
Evariste is an AI-driven Drug Discovery company
Patient-First Treatment
Evariste identifies and validates novel biomarkers to customize treatment to the patient
AI-Optimized Design
Evariste’s algorithmic modeling designs higher quality, differentiated molecules
Highly Automated R&D
Evariste has automated preclinical drug discovery enabling unprecedented scale and efficiency
Frobenius is Evariste's AI-enabled small molecule drug discovery platform
Using Frobenius, we are able to de-risk and progress projects with unrivaled efficiency
Each stage of our platform has been validated in challenging internal and collaborative projects
Frobenius Target
At Evariste we have developed the novel concept of synthetic lethal gene signatures, small panels of multi-omic biomarkers which reveal untargeted synthetic lethal interactions together with the patient population for which drugs targeting these genes will be most effective.
Our AI platform applies proprietary algorithms to large multi-omic biological and chemical datasets, including proprietary and public data. Targets are validated in disease-relevant models to ensure their clinical applicability. We identify specific predictive biomarkers or novel synthetic lethalities to ensure that the right patients will receive our treatments.
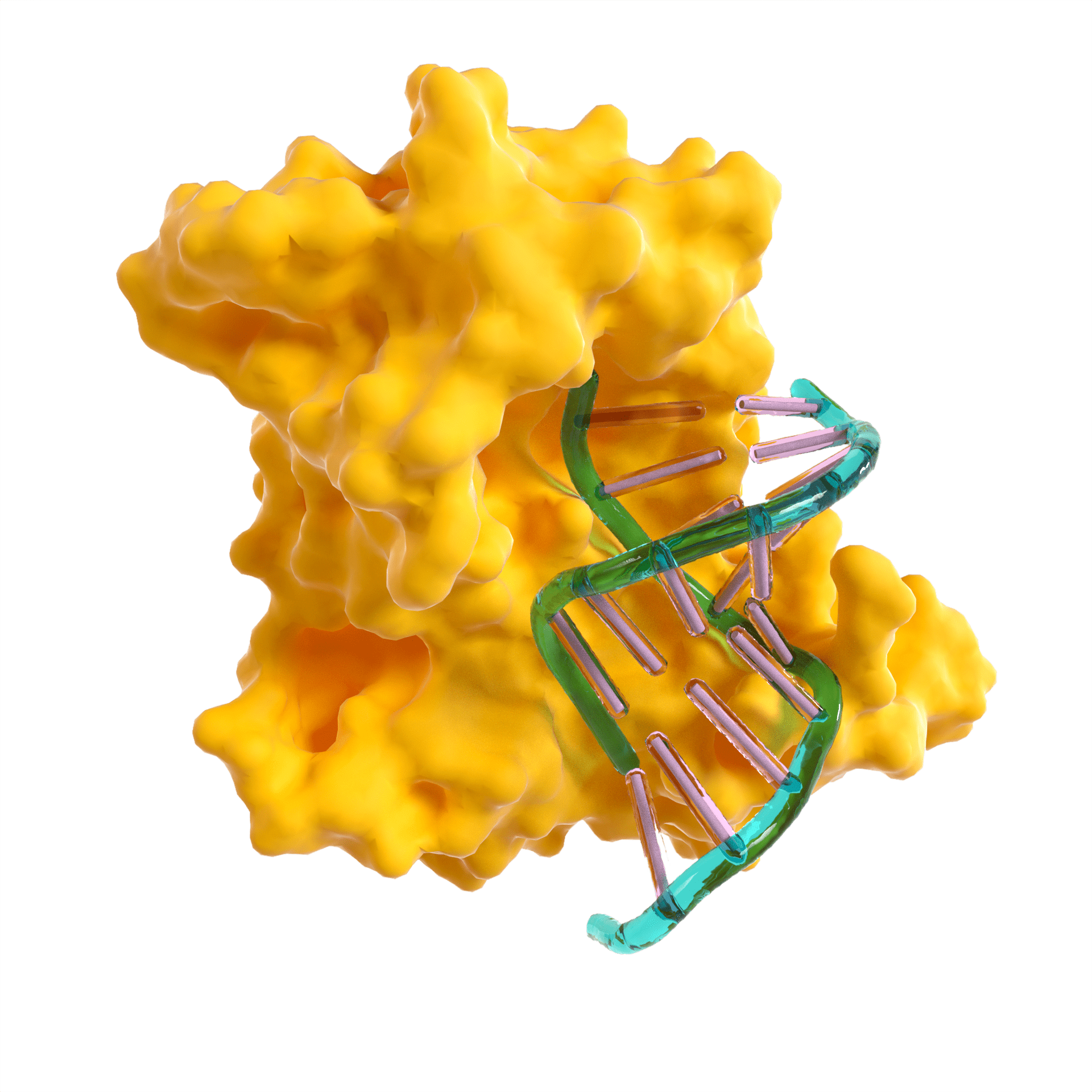
Frobenius Discovery
To translate the insights derived from Frobenius Target, Frobenius Discovery utilises information-rich hit finding tools to maximise the speed and success of ligand discovery.
We search ultra large libraries for hit-like chemical matter using statistically robust proprietary scoring functions. Probabilistic algorithms exploit the structure of combinatorial libraries, and facilitate virtual screening of libraries consisting of trillions of diverse compounds. The output of our screen is a diverse set of compounds in energetically favorable conformations hyper-enriched in key protein-ligand interactions.
We have successfully applied covalent fragment screens to find novel hit matter for previously undrugged protein families and target classes. The vast quantities of data generated by this technique maximises the speed of downstream development.

Frobenius Candidate
Frobenius Candidate designs and scores synthetically accessible molecules on a scale that would not be feasible for a team of chemists. Probabilistic modeling allows for principled combination of multiple endpoints, facilitating multi-parameter optimization. Our machine learning models are ideally suited to drug discovery as they are designed to work on small, noisy datasets.
The platform stands out not just for its ability to design synthetically accessible molecules, but also for its efficiency and efficacy. Through advanced statistical compound selection techniques, the platform consistently achieves a 10-fold increase in potency for every set of 30 compounds tested. Furthermore, in fewer than 50 compounds it has designed compounds with best-in-class selectivity against targets that are highly homologous. This level of precision and rapid optimization positions the Frobenius platform at the forefront of drug discovery, offering a transformative approach in an era where fast and efficient compound development is paramount.
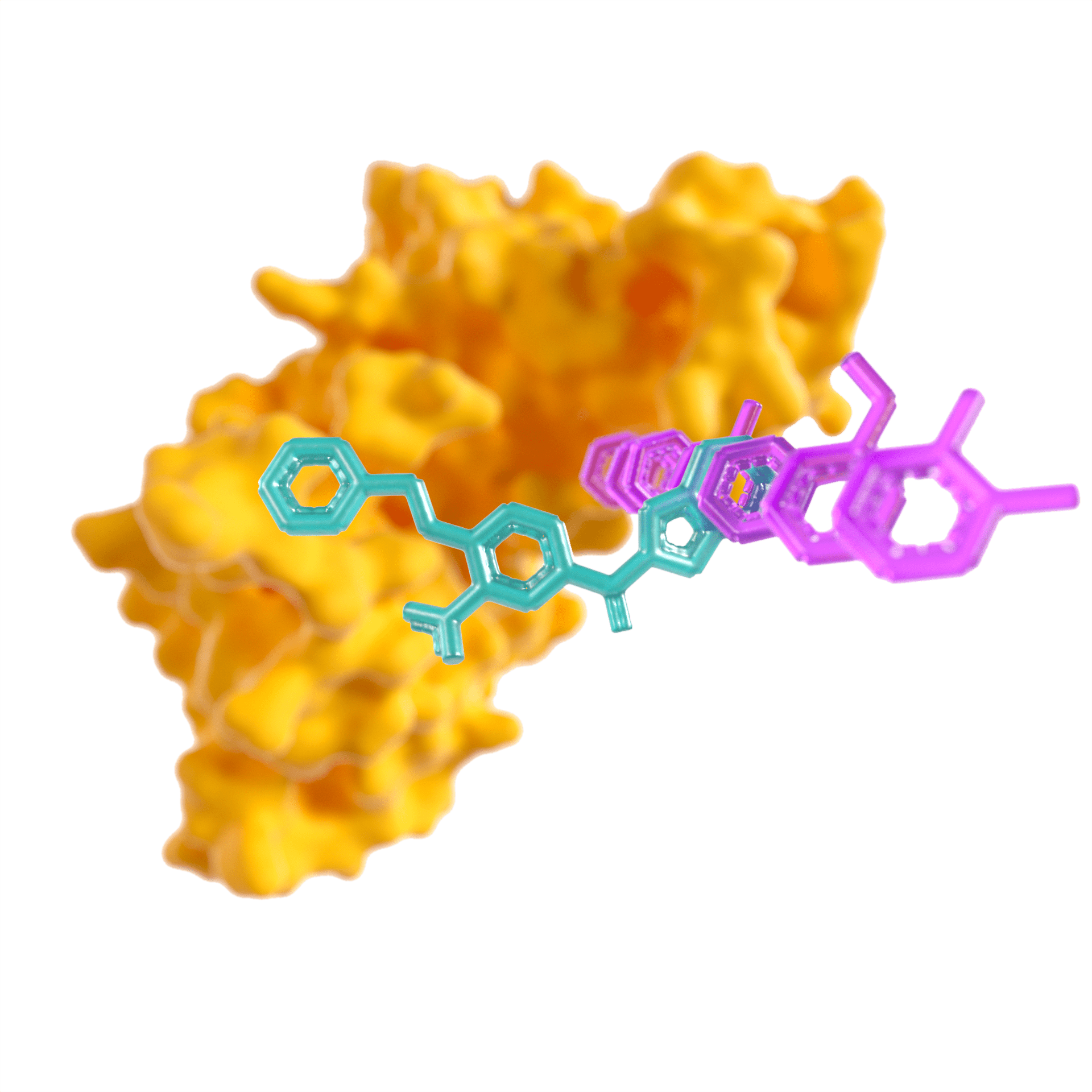
Using Frobenius, we have developed a pipeline of precision oncology therapeutics
This includes best-in-class inhibitors of validated synthetic lethal targets expanded to new indications, and first-in-class inhibitors of novel targets
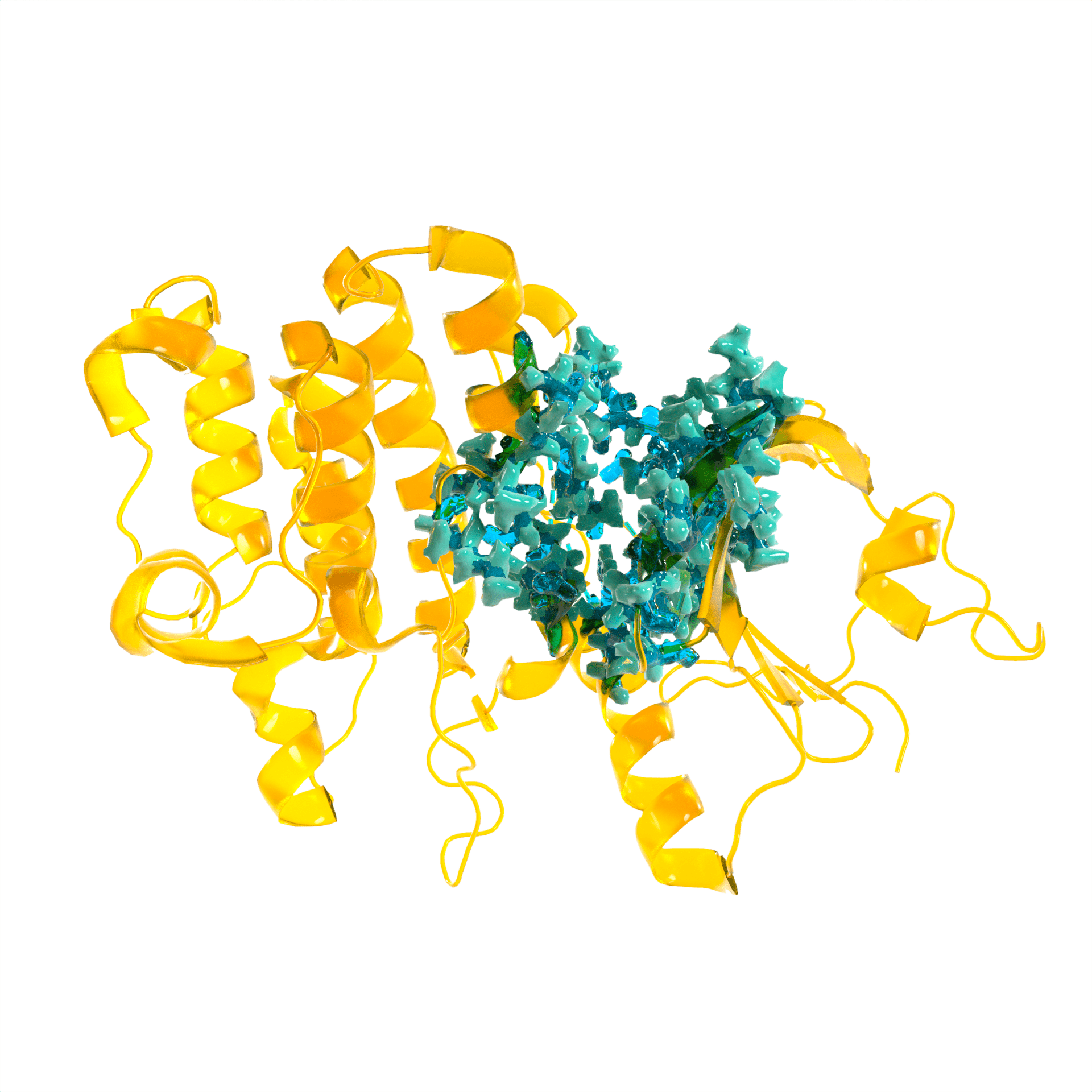
PKMYT1
Targeting PKMYT1 drives synthetic lethality in CCNE1 over-expressing cell lines, with clinical trials ongoing to explore this hypothesis. We have identified a novel biomarker for PKMYT1 synthetic lethality with potential for a significantly expanded patient population, and designed novel inhibitors with high potency and selectivity.
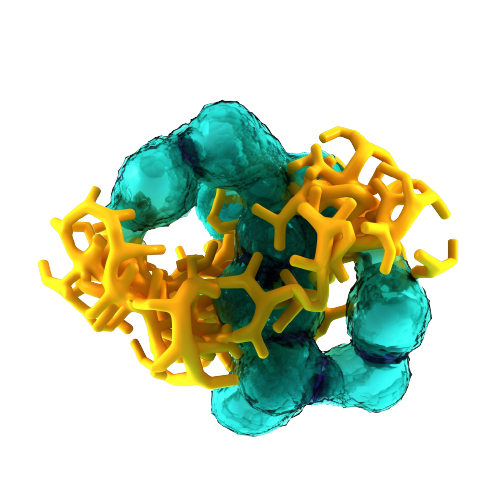
Undisclosed - Haematological Malignancies
Undisclosed target for heavily pretreated hematological malignancies. We have identified a new synthetic lethal target in a subset of acute myeloid leukemia and diffuse large B-cell lymphoma patients. This target is extremely novel and no enzymes of this class have been targeted with small molecule inhibitors or biologics.
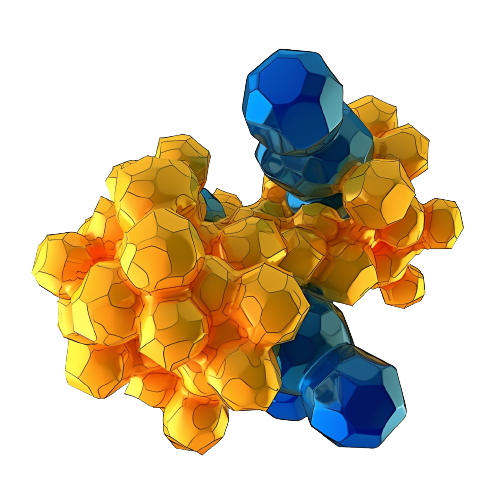
Undisclosed - Synthetic Lethal
A well-validated target that is currently in clinical trials without a clear biomarker strategy. We have identified a novel synthetic lethal partner to allow rapid patient selection and have designed inhibitors with best-in-class potency and selectivity.
Discovery
Optimization
Enabling
Anna graduated pre-med from Northwestern University as a neuroscience major. Post pre-med, she studied computer science. She previously helped businesses implement digital tools at a tech consulting company. She now applies her background knowledge in both computer science, business and science to run Evariste.
.png)
Anna Hercot
CHIEF EXECUTIVE OFFICER
Alfie received a PhD in Medicinal Chemistry from Newcastle University, where he worked on fragment based drug discovery. He then moved to the Institute of Cancer Research, working on lead optimization of PPI inhibitors. Alfie has worked on a variety of targets including kinases, bromodomains, sulfatases, PPIs, and E3 ligases.
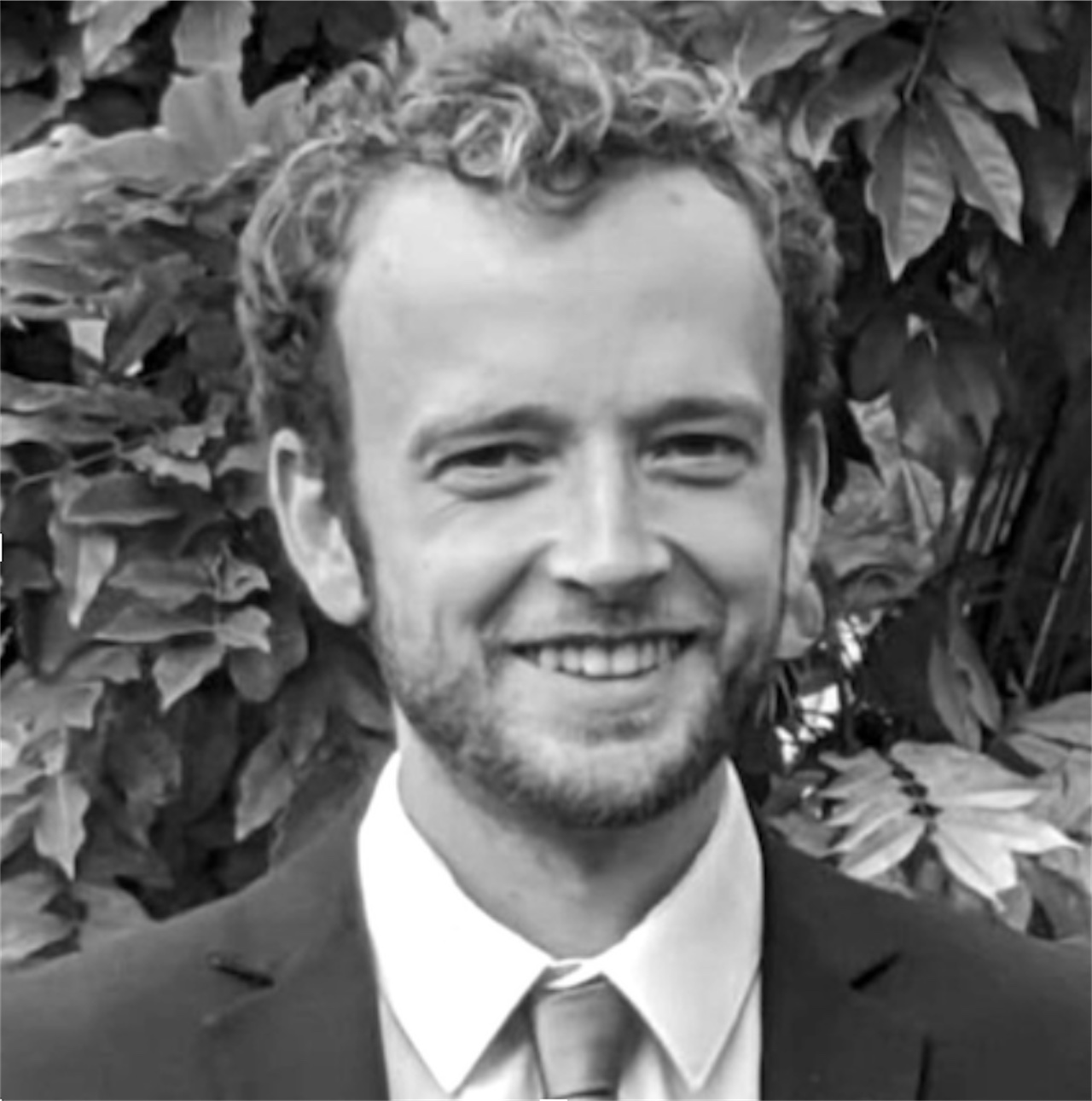
Alfie Brennan
CHIEF SCIENTIFIC OFFICER
Oliver studied for his Mathematics PhD at Oxford. He worked on developing new data analytic tools to describe the topology and geometry of data sets with interesting spatial structure, as well as the asymptotic topological properties of random simplicial complexes. Oliver develops statistically robust models applied to drug discovery.
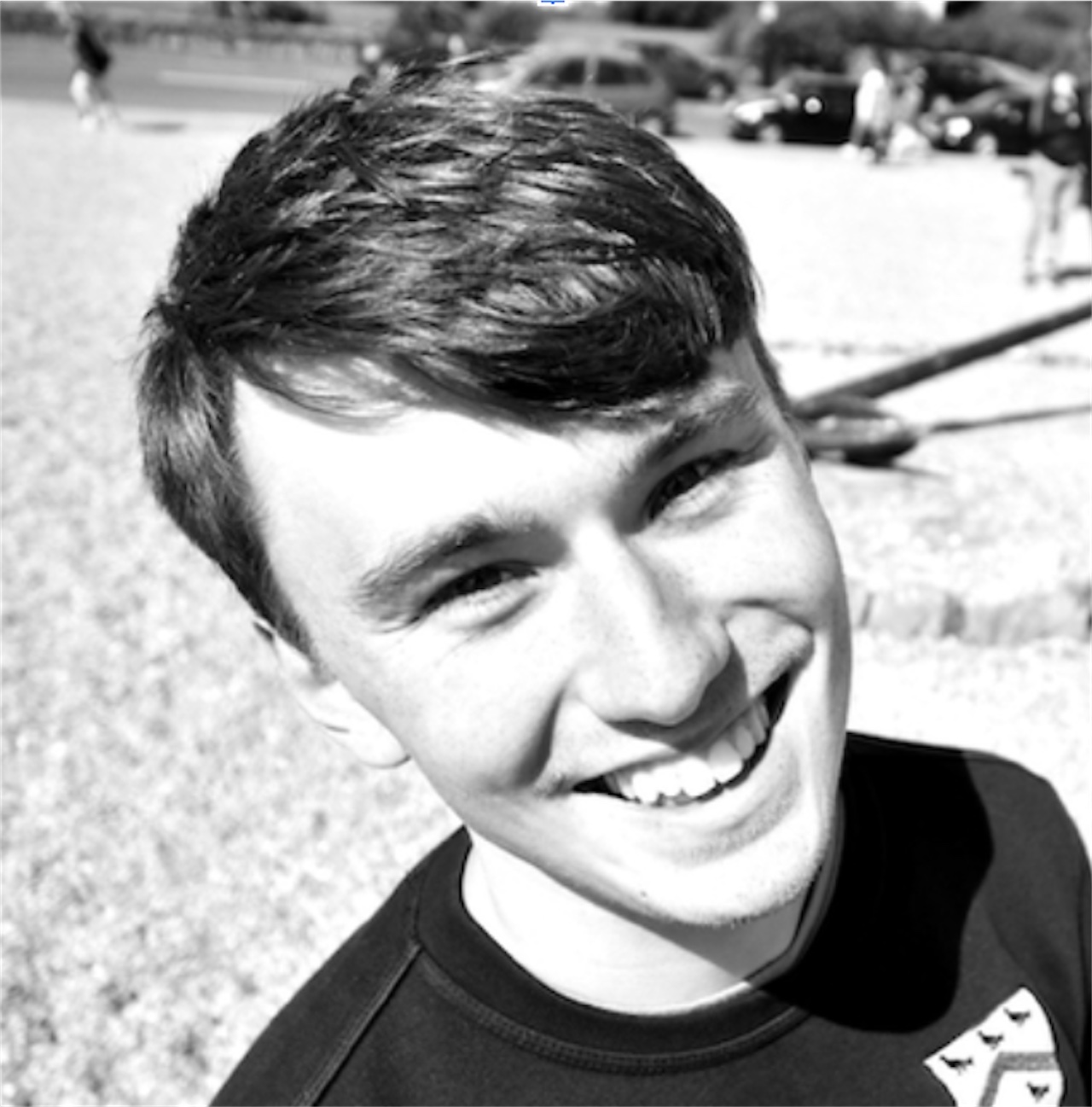
Oliver Vipond
HEAD QUANT
Ollie Watson received his PhD in Number Theory from University of Pennsylvania and has since worked in finance at D. E. Shaw and Tudor Capital, where he led a quantitative research team. He is an expert on optimization and statistical modeling in low signal-to-noise environments, and has taught courses on fitting predictive Bayesian models on noisy data.
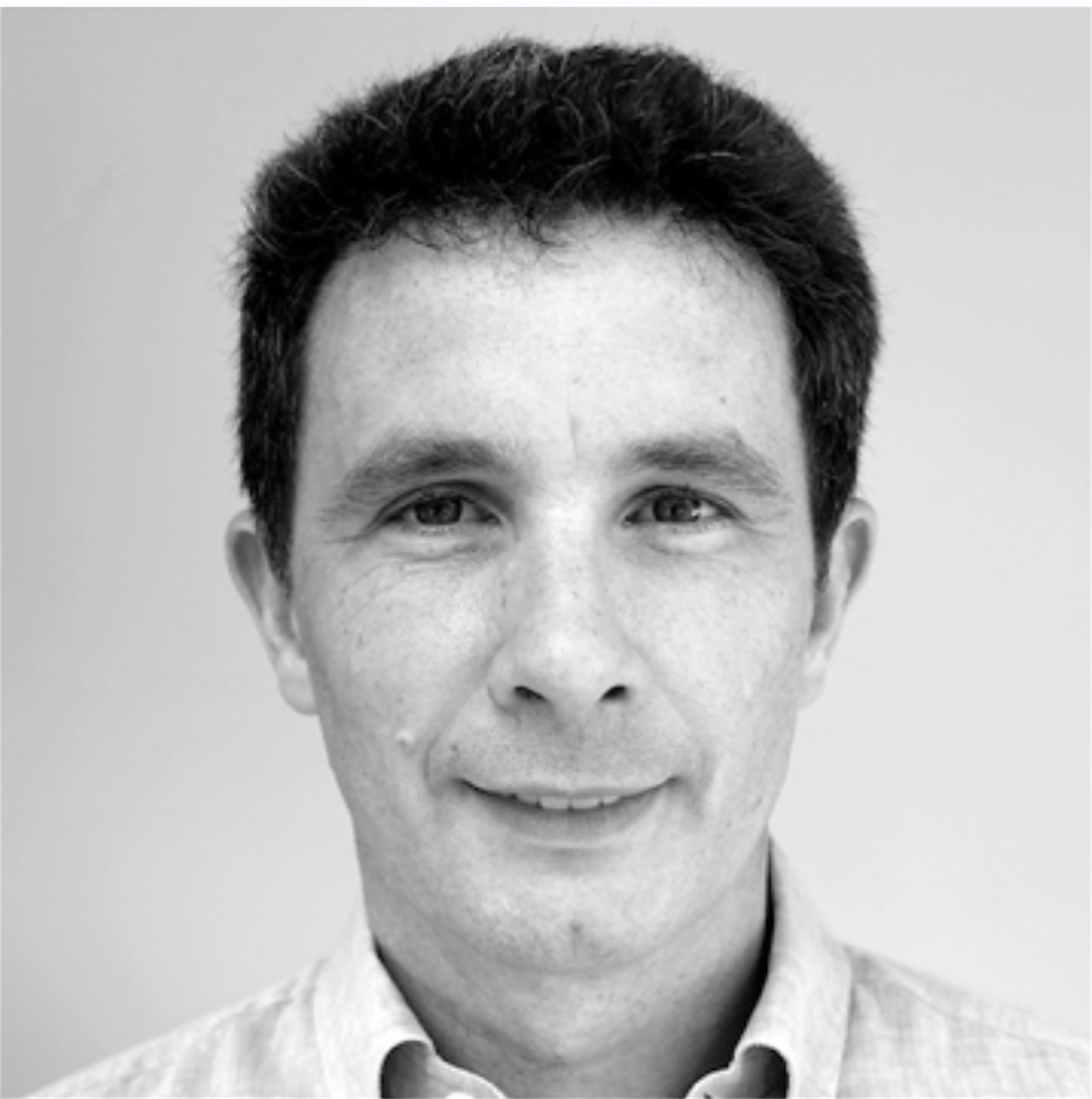
Oliver Watson
CHAIRMAN
Partnerships
We work in collaboration with biotech companies to accelerate the development of small molecules for a range of target classes in diverse disease areas. Our AI platform is able to support and advance discovery at every stage of preclinical development.
For any collaboration or business development inquiries:
Our Partners
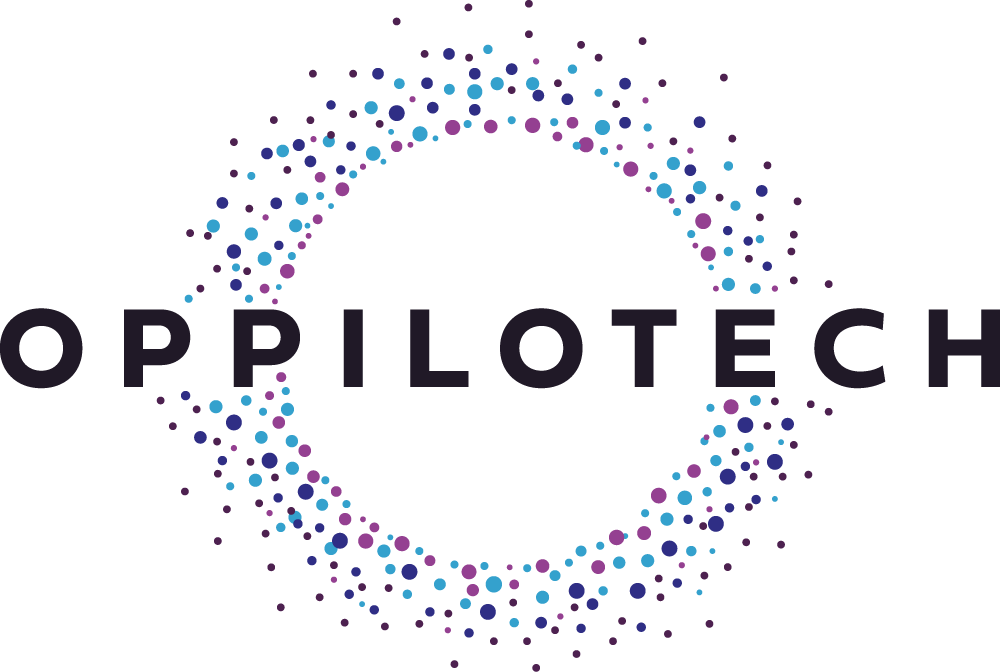
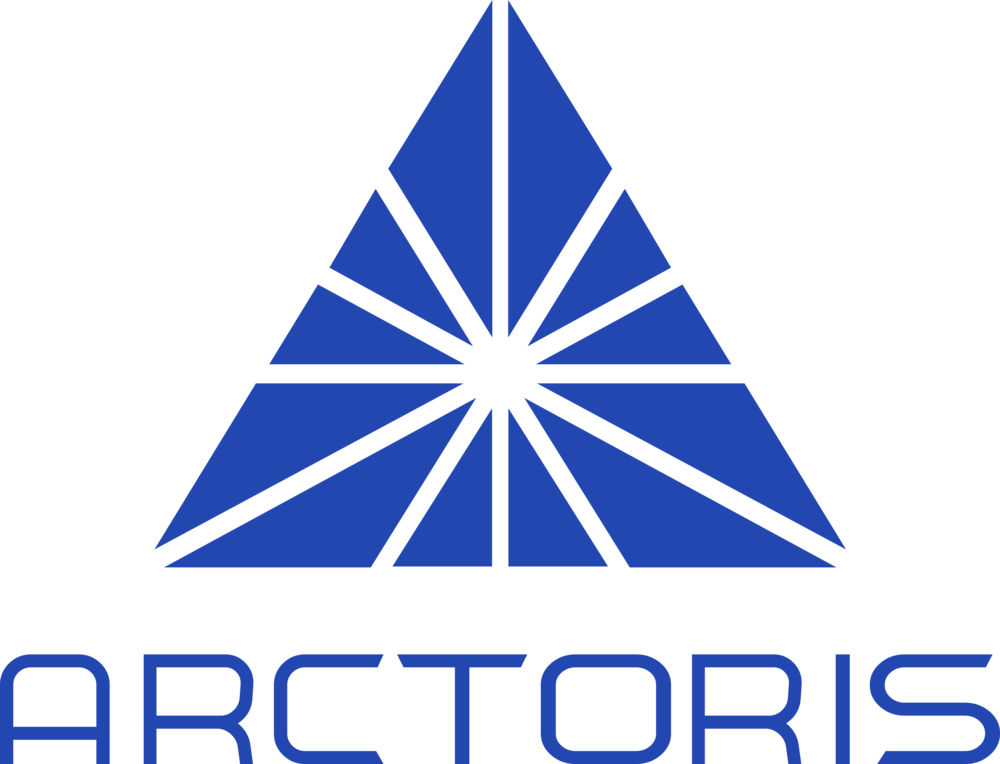
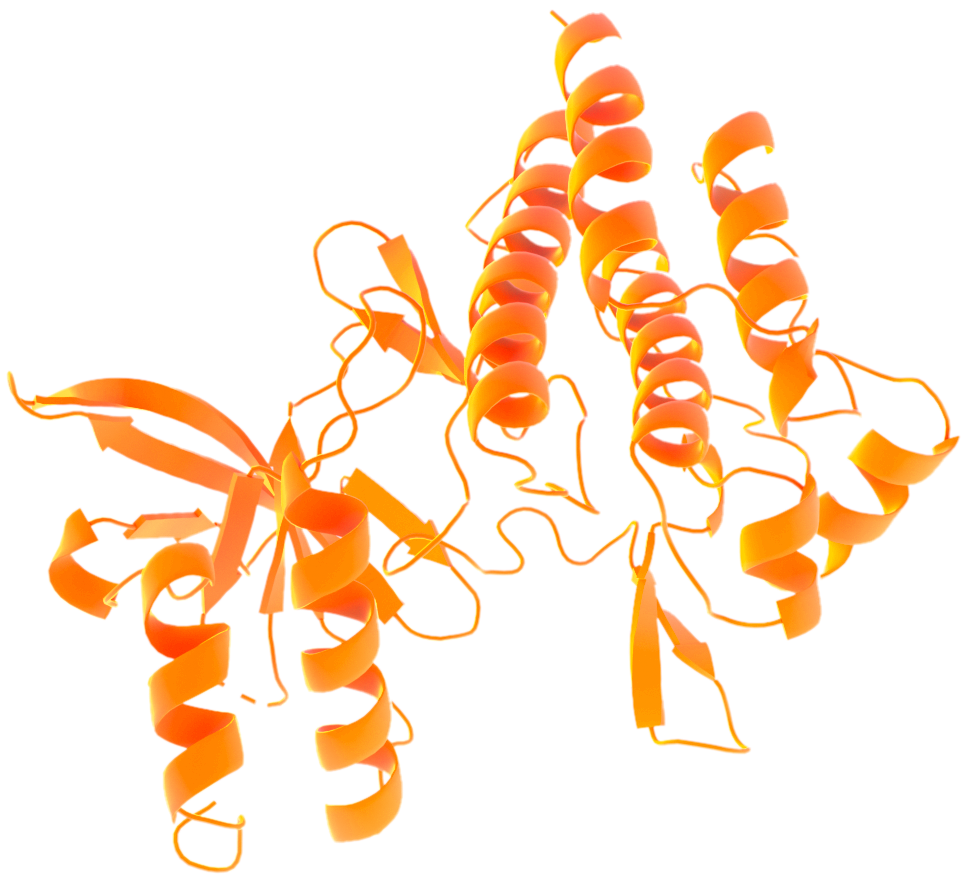
Contact us
News & updates
Stay updated with Evariste's blogposts